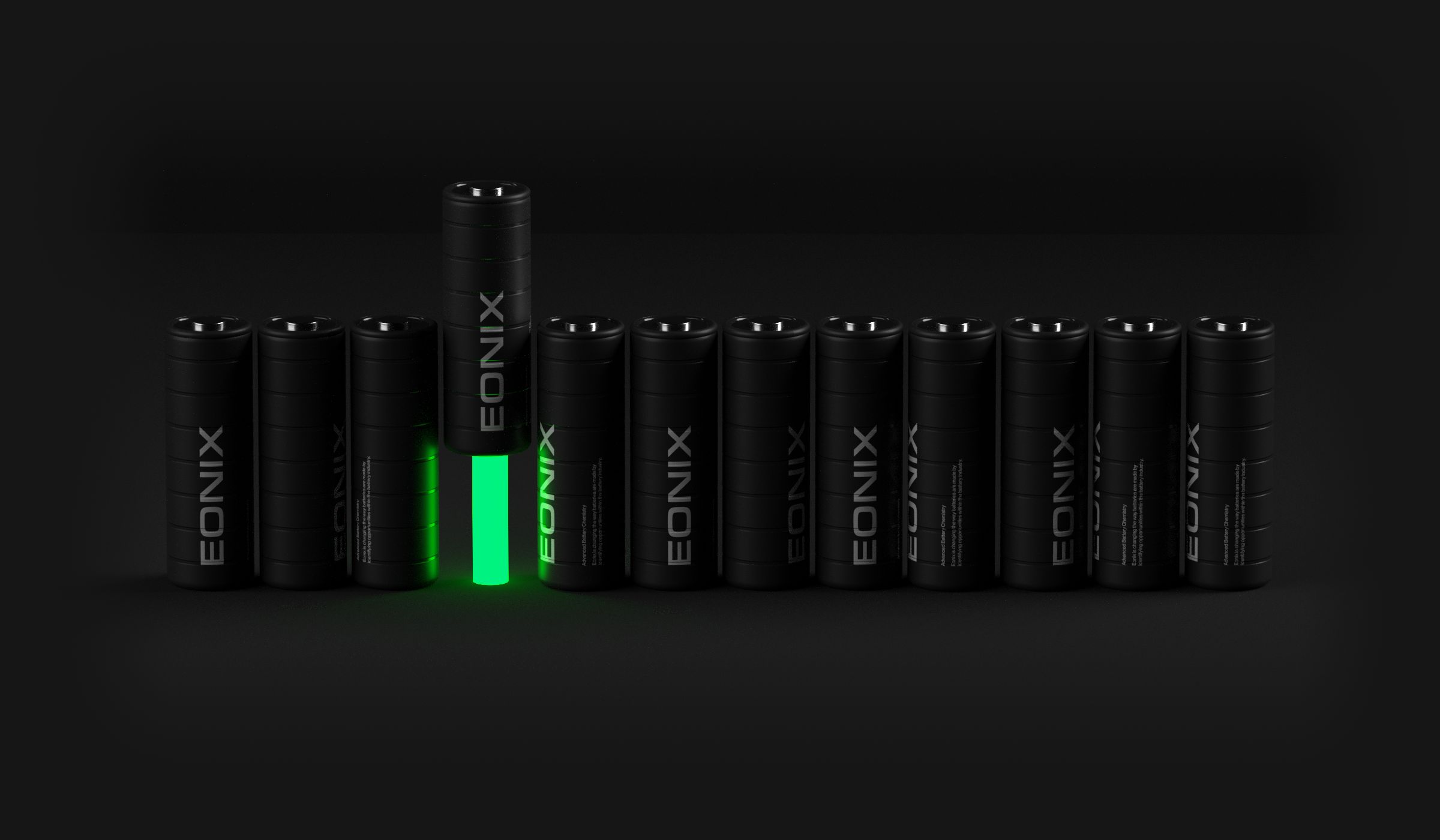
Designing Materials that Actually Make it into Batteries
Next-Gen Instruments & Techniques
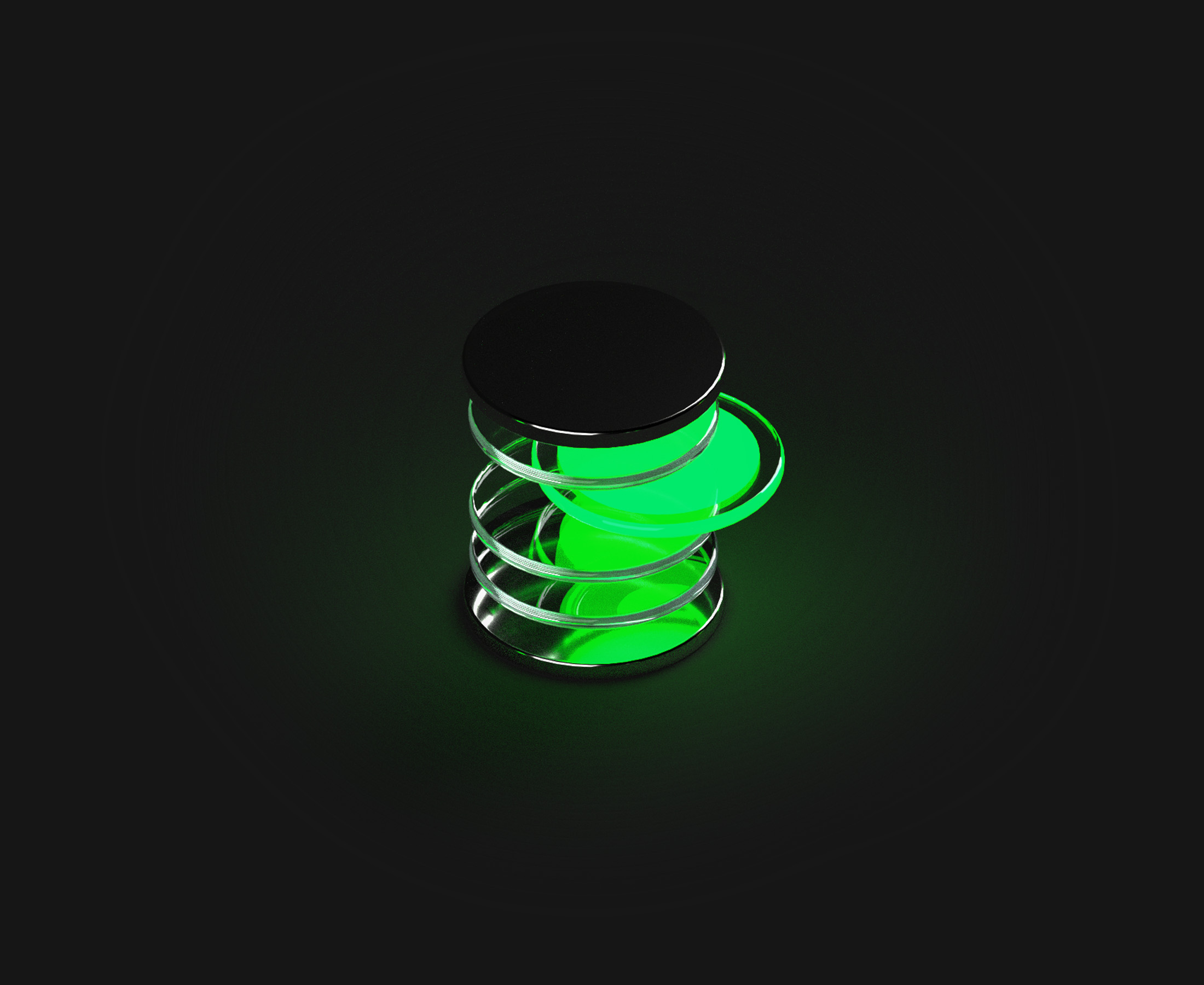
Directly measure materials, in real time
Eonix’s novel pseudo-reference electrode enables the full cell performance to be measured concurrently with the anode, cathode & electrolyte electrochemical characteristics without changing device behavior. This allows reactions to be explicitly observed in a real Li-ion battery providing significantly more data to build structure activity relationships and eliminating the need for tedious post-mortem characteristics.

Immediate Insights Into Material Behavior
Eonix’s auto analysis scripts immediately process the over 1 gigabyte of data generated per cell to calculate 15 unique cell features including SEI & CEI formation kinetics to elucidate the impact of new materials. The results are accessible through an interactive dashboard for quick review, with points of failure or advantages easily identifiable.

Automatically Generate Candidate Materials
Eonix’s physiochemical preparation and measurement systems are robotically assisted to generate a plethora of prospective candidates faster than any other lab effortlessly. Preparing solutions in gloveboxes is tedious and inaccurate, our lab enables batches of multi-component candidate solutions to be prepared rapidly & accurately from the comfort of a desktop.

Simulate Molecule & Solution Properties
Leveraging our partnership with Schrodinger, the leader in computational chemistry with the most accurate quantum mechanics & molecular dynamics simulations, Eonix is able to calculate the redox potential, dielectric constant, diffusion coefficient, solubility, and electric field density distribution of candidates before even stepping into the lab.
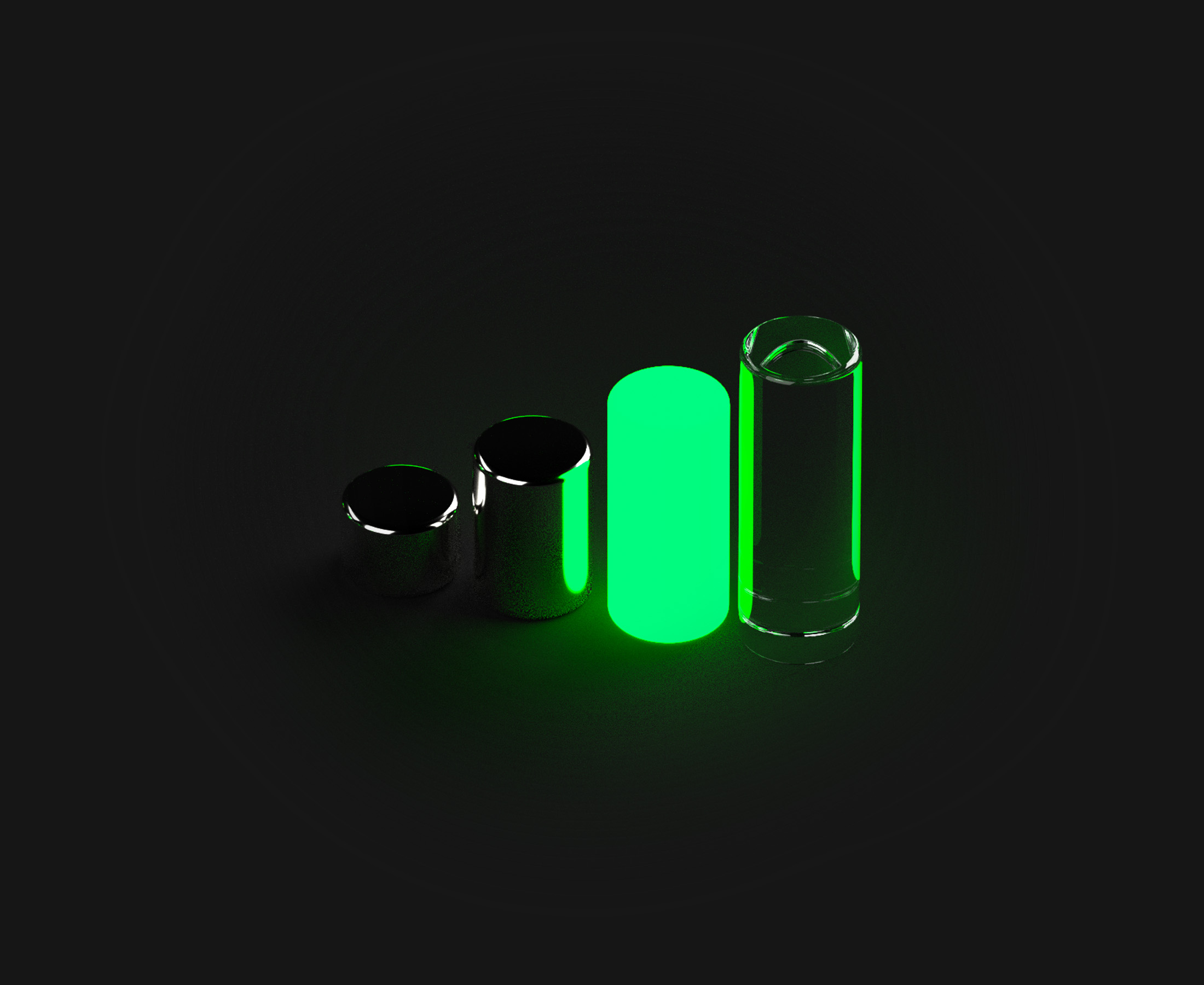
Predict Performance from Material Properties
Continuing to leverage our partnership with Schrodinger, Eonix is training deep learning models to not only predict solution properties, but battery performance. By coupling calculated electrolyte properties with unique physics based featurization and the comprehensive data generated from ATLAS, Eonix is training the most accurate model to predict battery performance of new materials enabling materials to be selected even faster.
The Future of Battery Design is Here
ATLAS data input
The existing library of ATLAS data is leveraged to identify initial problem-specific target properties from known structure activity relationships.
Future development
With ATLAS Gen 2, we will be able to input market specific needs, such as high temp, cycle life, andcost, into a De Novo Deep Learning Model to immediately generate best in class candidates.
Materials selection
Using physics simulations and deep learning models single molecule, solution & battery properties are calculated.
Top candidates are down selected from a library of millions of chemicals
Powered through partnership: physics simulations & deep learning models
Schrodinger’s Materials Suite has the most accurate quantum mechanics & molecular dynamics simulations available enabling quantitative analysis of complex electrolyte solutions.
Schrodinger’s Deep Learning QSAR & Cheminformatics platform enables high accuracy screening of large chemical libraries by using active learning to train models using datasets calculated from physics simulations.
Solution preparation + evaluation
Candidate solutions are robotically prepared.
ATLAS measures 15 unique battery properties generating 1GB of data per cell.
Future development
With ATLAS Gen 2, we will be able to input market specific needs, such as high temp, cycle life, andcost, into a De Novo Deep Learning Model to immediately generate best in class candidates.
Feedback cycle
The failure mechanisms are automatically calculated from ATLAS data.
An interactive dashboard report is generated.
Data populates a 2nd gen database structured to retrain deep learning models.
Plug n’ play result
Industrially available compounds with the desired characteristics are formulated.
Materials are plug n' play compatible with cell manufactured today for instant scalability.